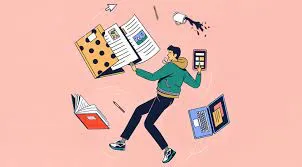
Statistical analysis serves as a vital foundation for Social and Behavioral Science (SBS) studies, enabling researchers to explore complex human behaviors, societal trends, and cultural dynamics. By using a variety of statistical techniques, researchers can interpret data, identify relationships, and draw meaningful conclusions that influence theory and practice. This article delves into the essential statistical techniques used in SBS studies, highlighting their applications and significance in advancing the field.
The Importance of Statistical Analysis in SBS Studies
SBS studies often address questions related to human behavior, social structures, and cultural interactions. These inquiries frequently involve large datasets and multifaceted variables, making statistical analysis a crucial tool for distilling information and deriving actionable insights.
For example, understanding the effects of socioeconomic status on mental health requires analyzing diverse data points such as income levels, education, employment status, and access to healthcare. Without robust statistical methods, uncovering meaningful patterns and correlations in such data would be unfeasible.
Descriptive Statistics: The Foundation of Data Interpretation
Descriptive statistics are the starting point for most sbs studies, providing a summary of data through measures like mean, median, mode, variance, and standard deviation. These metrics help researchers understand the central tendencies and variability within their datasets.
For instance, in a study examining public attitudes toward climate change, descriptive statistics might reveal the average level of concern across different demographics. Visual tools like histograms, pie charts, and box plots further enhance data interpretation, making results accessible to wider audiences.
Inferential Statistics: Drawing Conclusions from Data
While descriptive statistics summarize data, inferential statistics enable researchers to generalize findings from a sample to a larger population. Techniques such as hypothesis testing, confidence intervals, and significance tests are essential for this purpose.
Consider an SBS study investigating the impact of remote work on employee productivity. Inferential statistics can help determine whether observed differences in productivity are statistically significant or simply due to chance. By applying these methods, researchers can make data-driven predictions and recommendations.
Regression Analysis: Exploring Relationships Between Variables
Regression analysis is one of the most commonly used techniques in SBS studies for examining relationships between dependent and independent variables. This method helps researchers quantify the effect of one variable on another while controlling for confounding factors.
For example, multiple regression analysis can assess how education, income, and age simultaneously influence voting behavior. Advanced techniques like logistic regression are also employed when dealing with binary outcomes, such as whether someone is likely to adopt a new technology.
Multivariate Techniques: Tackling Complex Data
SBS studies often involve datasets with numerous variables, necessitating the use of multivariate statistical techniques. Methods like factor analysis, cluster analysis, and structural equation modeling (SEM) allow researchers to analyze interrelated variables simultaneously.
Factor Analysis: Used to identify underlying constructs from observed variables, such as discovering latent factors influencing job satisfaction.
Cluster Analysis: Groups individuals or items into clusters based on similarities, which can help segment populations for targeted interventions.
SEM: Combines multiple statistical methods to test theoretical models, ideal for exploring complex relationships in social science research.
These techniques provide a deeper understanding of the multifaceted nature of human behavior and societal interactions.
Challenges and Best Practices in Statistical Analysis
Despite its benefits, conducting statistical analysis in SBS studies comes with challenges. Issues such as missing data, sample bias, and measurement errors can compromise the validity of findings. Researchers must carefully design studies, select appropriate techniques, and ensure ethical data handling to mitigate these challenges.
Additionally, interpreting statistical results requires not only technical expertise but also a deep understanding of the subject matter. Misinterpretation of data can lead to flawed conclusions, underscoring the importance of combining statistical skills with domain knowledge.
Conclusion: Empowering SBS Studies with Statistical Analysis
Statistical analysis is a cornerstone of SBS studies, offering powerful tools to explore, interpret, and present complex data. From descriptive and inferential statistics to advanced multivariate techniques, these methods enable researchers to uncover patterns, test hypotheses, and inform decisions.
As technology continues to advance, the capabilities of statistical analysis in SBS research will expand, providing even more sophisticated tools for tackling the intricacies of social and behavioral phenomena. By mastering these techniques, researchers can make meaningful contributions to their fields and address the pressing challenges of our time.